FeiYuePsych2012Project: Difference between revisions
imported>Psych2012 |
imported>Psych2012 |
||
(33 intermediate revisions by the same user not shown) | |||
Line 3: | Line 3: | ||
In today's computer graphics, a large amount of textures are mapped onto surfaces of objects to add visual details and provide realism. These textures can consume a large portion of system and video memory. An efficient compression algorithm can reduce the amount of memory required for storing the textures and allow better performance by reducing the memory bandwidth used to fetch textures. An efficient real-time compression algorithm is important for dynamic texture generation and for texture streaming where the source compression format is different than the GPU hardware compression format. | In today's computer graphics, a large amount of textures are mapped onto surfaces of objects to add visual details and provide realism. These textures can consume a large portion of system and video memory. An efficient compression algorithm can reduce the amount of memory required for storing the textures and allow better performance by reducing the memory bandwidth used to fetch textures. An efficient real-time compression algorithm is important for dynamic texture generation and for texture streaming where the source compression format is different than the GPU hardware compression format. | ||
The DXT compression format is common across most major GPU hardware. DXT1, stores 16 input pixels in 64 bits of output. The output consists of two 16-bit RGB 5:6:5 color values and a 4x4 two bit lookup table | The DXT compression format is common across most major GPU hardware. DXT1, stores 16 input pixels in 64 bits of output. The output consists of two 16-bit RGB 5:6:5 color values and a 4x4 two bit lookup table [1]. The compression ratio is 6:1 for 24 bit source images. DXT5, converts 16 input pixels into 128 bits of output. The output consists of 64 bits of alpha channel data, followed by 64 bits of color data [1]. The compression ratio is 4:1. | ||
The DXT compression format loses some image quality and introduces blocking artifacts. Several attempts have been made to improve image quality for this format. The state-of-art in real-time DXT compression is "Real-Time YCoCg-DXT compression" []. | == Real-Time YCoCg-DXT Compression == | ||
The DXT compression format loses some image quality and introduces blocking artifacts. Several attempts have been made to improve image quality for this format. The state-of-art in real-time DXT compression is "Real-Time YCoCg-DXT compression" [2]. This algorithm works in the YCoCg space, which represents the luma (Y), orange chroma (Co), and green chroma (Cg) components. The YCoCg space can be encoded similar to YCbCr, but it is invented for better compression of the RGB space. It is possible to losslessly transform from RGB to YCoCg when using 2 more bits for YCoCg representation than for RGB [3]. | |||
The YCoCg-DXT compression first converts from RGB to YCoCg. Then, it operates on every 4x4 pixel blocks. The algorithm finds the maximum and minimum values from each of the Y, Co, and Cg channels. These values are to be stored during the encoding process. The algorithm calculates six intermediate values for the Y channel, and two for the color channels. Each pixel of the 4x4 block is quantized to the calculated values. For the color channel, two combinations of max/min values exist: max Co to min Cg, or max Cg to min Co. The algorithm determines which of the two combination provides better results for quantization. | |||
The | The YCoCg-DXT compression algorithm can increase the RGB-PSNR by 6dB or more [2], there are other steps one could take to further improve the image quality. This project focuses on three additional steps to reduce compression artifacts on top of the Real-Time YCoCg-DXT Compression algorithm. The artifacts we want to reduce in this effort are banding and blocking. As well, we want to address the loss of details in images after compression. | ||
= Methods = | = Methods = | ||
The algorithm | The base algorithm in this project is the YCoCg-DXT compression algorithm. The entire pipeline of this algorithm is implemented in code for comparison and evaluation purposes. On top of that, three new operations are added to the pipeline to make the improvements. These operations are described below. | ||
The first step after transforming from RGB to YCoCg space is to do a non-linear remap of the chroma components after mapping Co and Cg to the range of -1 to 1. Using the function described in | The first step after transforming from RGB to YCoCg space is to do a non-linear remap of the chroma components after mapping Co and Cg to the range of -1 to 1. Using the function described in the equation below, we expand the near zero region of the chroma value to increase precision in less saturated colors, at the expense of a reduction of precision in the more saturated colors. This is to take advantage of the property of the human vision system in that the eye is more sensitive to chroma differences in less saturated colors compared to more saturated colors. The effects of the equation below is illustrated by Figure 1. | ||
Co_out = sqrt(abs(Co_in))*sign(Co_in) | |||
1 2 1; | [[File:chromaRemap.png |thumb|center|537px| Figure 1: Non-Linear Remap of Chroma]] | ||
The second step is to apply sharpening to the image. Sharpening is done by adding a scaled amount of the difference between the pixel and a lowpass filter applied to the pixel. The lowpass kernel is described in the equation below. The sharpening is only done to the luma (Y) channel, and is limited to one Y quantization level (-/+ 1.0/512.0). This effort is to shape some of the quantization artifacts on the image such that detail is not lost. | |||
[1 2 1; | |||
2 4 2; | 2 4 2; | ||
1 2 1 | 1 2 1] | ||
The third step is to add noise to each of the Y, Co and Cg channels. This would affect the least significant bits during encoding, therefore reducing the banding effects. Less noise is added to the Cg channel than the Co, and even less to the Y channel. This is because the human eye is more sensitive to the luma and the green chroma channels. Scaling is as follows, | The third step is to add noise to each of the Y, Co and Cg channels. This would affect the least significant bits during encoding, therefore reducing the banding effects. Less noise is added to the Cg channel than the Co, and even less to the Y channel. This is because the human eye is more sensitive to the luma and the green chroma channels. Scaling is as follows, | ||
Y -> +/- 1.0/2048.0 | Y -> +/- 1.0/2048.0 | ||
Co -> +/- 1.0/128.0 | Co -> +/- 1.0/128.0 | ||
Cg -> +/- 1.0/256.0 | Cg -> +/- 1.0/256.0 | ||
Lastly, quantization is applied, and the image is encoded and decoded to complete the compression pipeline. | After these steps, the rest of the steps to complete the compression are the same as the Real-time YCoCg-DXT5 algorithm. For each 4x4 blocks, the maximum and minimum values for each of the Y, Co and Cg channels are determined. Lastly, quantization is applied, and the image is encoded and decoded to complete the compression pipeline. | ||
= Results = | = Results = | ||
We used screen shots from current-generation games, and compare visual results between the Real-Time YCoCg-DXT compression algorithm and our algorithm. The results are broken down into three distinct areas as follows. | |||
== Retention of Image Details == | |||
== | The following set of example illustrate the effect of the micro-sharpening operation. Figure 2 shows the original picture, and we transformed the picture such that we can see the details better, shown in Figure 3. | ||
== | |||
[[File:A-origin.png |thumb|center|800px| Figure 2: Original Image ]] | |||
[[File:A-base.png |thumb|center|800px| Figure 3: Original Image, transformed]] | |||
Figure 4 shows a cropped version of the original picture, emphasizing on the wall details on the right hand side. In Figure 5, we applied the DXT5 compression method, losing all the details of the brick pattern on the right hand side wall. In Figure 6, our version of the algorithm retains the brick pattern. This is because of the micro-sharpening adjustments from our algorithm. | |||
[[File:A-base-crop.png |thumb|center|800px| Figure 4: Original Image, transformed, cropped ]] | |||
[[File:A-dxt5-crop.png |thumb|center|800px| Figure 5: Real-Time YCoCg-DXT Compression (DXT5), loss of details of brick pattern on right hand side]] | |||
[[File:A-fei-crop.png |thumb|center|800px| Figure 6: High Quality Real-Time DXT Compression (Our Method), details recovered on right hand side]] | |||
== Reduction of Blocking Artifacts == | |||
The following example set illustrates blocking artifacts. For the ease of viewing, we applied the same high contrast to all images to amplify the artifacts. We also magnified all the images with a 4x factor. The original image, shown in Figure 7, has no blocking artifacts nor significant noise. After the DXT5 compression (Figure 8), severe blocking can be seen. Our algorithm attempts to mitigate the problem through the noise dithering operation. In Figure 9, the blocking artifacts are reduced, at the expense of adding noise. | |||
[[File:A-1.png |thumb|center|800px| Figure 7: Original Image, no blocking artifacts, no noise]] | |||
[[File:A-2.png |thumb|center|800px| Figure 8: Real-Time YCoCg-DXT Compression (DXT5), heavy blocking artifacts]] | |||
[[File:A-3.png |thumb|center|800px| Figure 9: High Quality Real-Time DXT Compression (Our Method), blocking removed, but with noise]] | |||
== Removal of Banding == | |||
The following example set illustrates how banding is eliminated for the compressed image. Figure 10 is the original image. The sky consists of a smooth gradient with orange and yellow tints. After the DXT5 compression (Figure 11), banding can be seen from the orange and yellow part, and the gradient is no longer smooth. Using our method (Figure 12), the sky does not have any banding, and it looks identical to the original image. The removal of this artifact is contributed by the added precision in the chroma channels from the non-linear remap. | |||
[[File:Example1-base.png |thumb|center|800px| Figure 10: Original Image, no banding]] | |||
[[File:Example1-dxt5.png |thumb|center|800px| Figure 11: Real-Time YCoCg-DXT Compression (DXT5), with banding]] | |||
[[File:Example1-fei.png |thumb|center|800px| Figure 12: High Quality Real-Time DXT Compression (Our Method), banding removed]] | |||
= Conclusions = | = Conclusions = | ||
The improved algorithm effectively diminishes the banding and blocking artifacts caused by the traditional DXT5 algorithm through adding noise. The micro-sharpening adds back some fine details which are lost during the compression. And the non-linear remap minimizes some of the negative effects of the quantization. Overall, a higher quality image is produced without compromising the compression ratio. | |||
The next step is to extend the current work to a non-real-time compression algorithm. The current algorithm is suited for real-time, which has a strict performance constraint. An extension to non-real-time work would relax this constraint and allow a more elaborate algorithm that works for offline image processing. | |||
= References = | = References = | ||
[1] http://en.wikipedia.org/wiki/S3_Texture_Compression | |||
[2] http://developer.download.nvidia.com/whitepapers/2007/Real-Time-YCoCg-DXT-Compression/Real-Time%20YCoCg-DXT%20Compression.pdf | |||
[3] http://wiki.multimedia.cx/index.php?title=YCoCg | |||
= Appendix I - Code and Data = | = Appendix I - Code and Data = |
Latest revision as of 19:49, 21 March 2012
Background
In today's computer graphics, a large amount of textures are mapped onto surfaces of objects to add visual details and provide realism. These textures can consume a large portion of system and video memory. An efficient compression algorithm can reduce the amount of memory required for storing the textures and allow better performance by reducing the memory bandwidth used to fetch textures. An efficient real-time compression algorithm is important for dynamic texture generation and for texture streaming where the source compression format is different than the GPU hardware compression format.
The DXT compression format is common across most major GPU hardware. DXT1, stores 16 input pixels in 64 bits of output. The output consists of two 16-bit RGB 5:6:5 color values and a 4x4 two bit lookup table [1]. The compression ratio is 6:1 for 24 bit source images. DXT5, converts 16 input pixels into 128 bits of output. The output consists of 64 bits of alpha channel data, followed by 64 bits of color data [1]. The compression ratio is 4:1.
Real-Time YCoCg-DXT Compression
The DXT compression format loses some image quality and introduces blocking artifacts. Several attempts have been made to improve image quality for this format. The state-of-art in real-time DXT compression is "Real-Time YCoCg-DXT compression" [2]. This algorithm works in the YCoCg space, which represents the luma (Y), orange chroma (Co), and green chroma (Cg) components. The YCoCg space can be encoded similar to YCbCr, but it is invented for better compression of the RGB space. It is possible to losslessly transform from RGB to YCoCg when using 2 more bits for YCoCg representation than for RGB [3].
The YCoCg-DXT compression first converts from RGB to YCoCg. Then, it operates on every 4x4 pixel blocks. The algorithm finds the maximum and minimum values from each of the Y, Co, and Cg channels. These values are to be stored during the encoding process. The algorithm calculates six intermediate values for the Y channel, and two for the color channels. Each pixel of the 4x4 block is quantized to the calculated values. For the color channel, two combinations of max/min values exist: max Co to min Cg, or max Cg to min Co. The algorithm determines which of the two combination provides better results for quantization.
The YCoCg-DXT compression algorithm can increase the RGB-PSNR by 6dB or more [2], there are other steps one could take to further improve the image quality. This project focuses on three additional steps to reduce compression artifacts on top of the Real-Time YCoCg-DXT Compression algorithm. The artifacts we want to reduce in this effort are banding and blocking. As well, we want to address the loss of details in images after compression.
Methods
The base algorithm in this project is the YCoCg-DXT compression algorithm. The entire pipeline of this algorithm is implemented in code for comparison and evaluation purposes. On top of that, three new operations are added to the pipeline to make the improvements. These operations are described below.
The first step after transforming from RGB to YCoCg space is to do a non-linear remap of the chroma components after mapping Co and Cg to the range of -1 to 1. Using the function described in the equation below, we expand the near zero region of the chroma value to increase precision in less saturated colors, at the expense of a reduction of precision in the more saturated colors. This is to take advantage of the property of the human vision system in that the eye is more sensitive to chroma differences in less saturated colors compared to more saturated colors. The effects of the equation below is illustrated by Figure 1.
Co_out = sqrt(abs(Co_in))*sign(Co_in)
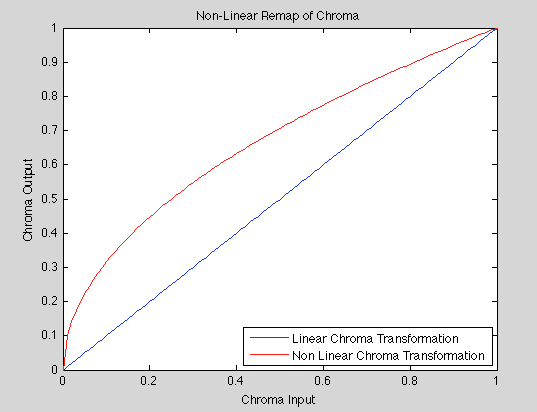
The second step is to apply sharpening to the image. Sharpening is done by adding a scaled amount of the difference between the pixel and a lowpass filter applied to the pixel. The lowpass kernel is described in the equation below. The sharpening is only done to the luma (Y) channel, and is limited to one Y quantization level (-/+ 1.0/512.0). This effort is to shape some of the quantization artifacts on the image such that detail is not lost.
[1 2 1;
2 4 2;
1 2 1]
The third step is to add noise to each of the Y, Co and Cg channels. This would affect the least significant bits during encoding, therefore reducing the banding effects. Less noise is added to the Cg channel than the Co, and even less to the Y channel. This is because the human eye is more sensitive to the luma and the green chroma channels. Scaling is as follows,
Y -> +/- 1.0/2048.0
Co -> +/- 1.0/128.0
Cg -> +/- 1.0/256.0
After these steps, the rest of the steps to complete the compression are the same as the Real-time YCoCg-DXT5 algorithm. For each 4x4 blocks, the maximum and minimum values for each of the Y, Co and Cg channels are determined. Lastly, quantization is applied, and the image is encoded and decoded to complete the compression pipeline.
Results
We used screen shots from current-generation games, and compare visual results between the Real-Time YCoCg-DXT compression algorithm and our algorithm. The results are broken down into three distinct areas as follows.
Retention of Image Details
The following set of example illustrate the effect of the micro-sharpening operation. Figure 2 shows the original picture, and we transformed the picture such that we can see the details better, shown in Figure 3.
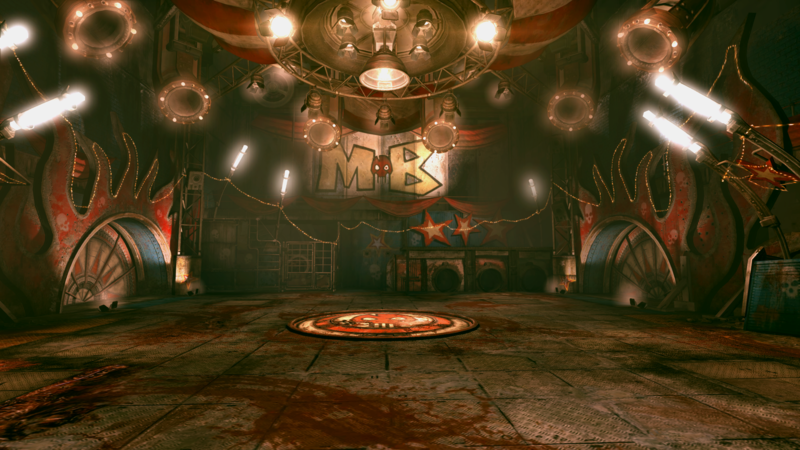
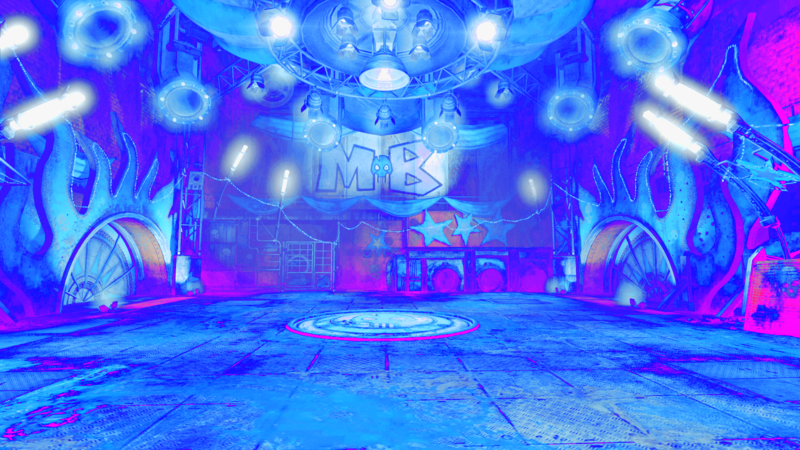
Figure 4 shows a cropped version of the original picture, emphasizing on the wall details on the right hand side. In Figure 5, we applied the DXT5 compression method, losing all the details of the brick pattern on the right hand side wall. In Figure 6, our version of the algorithm retains the brick pattern. This is because of the micro-sharpening adjustments from our algorithm.
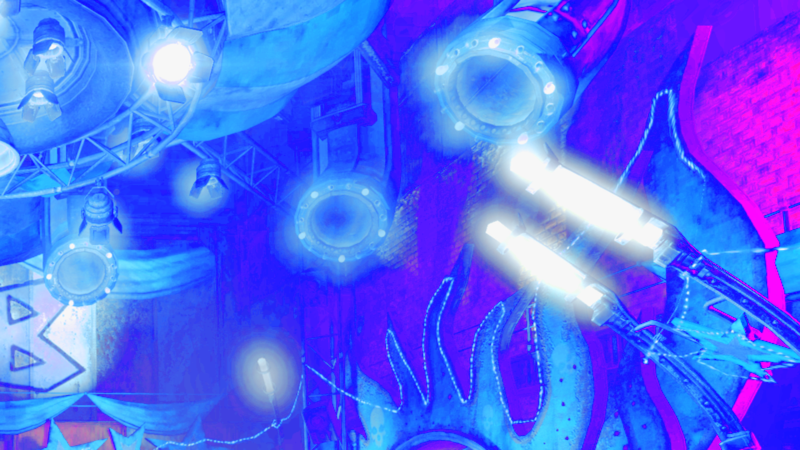
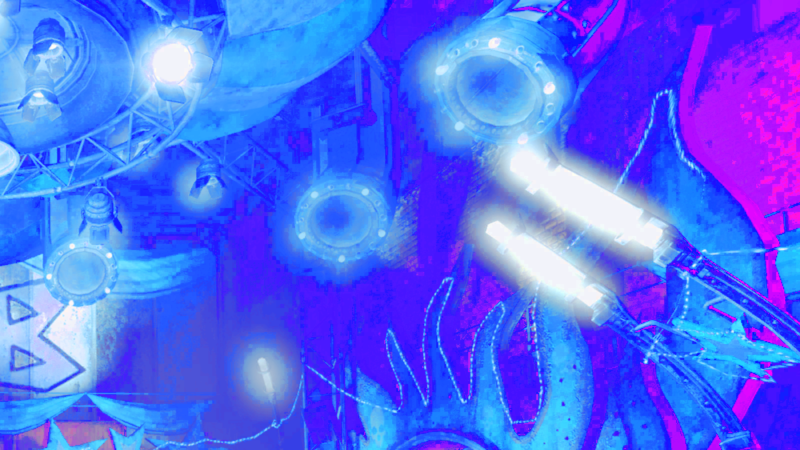
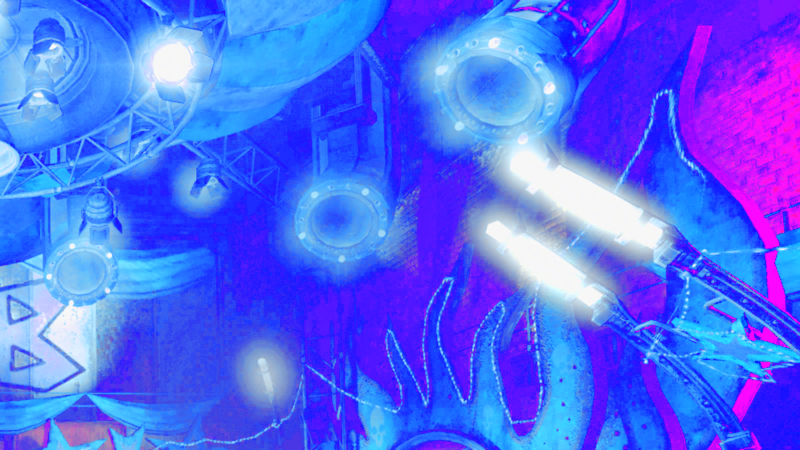
Reduction of Blocking Artifacts
The following example set illustrates blocking artifacts. For the ease of viewing, we applied the same high contrast to all images to amplify the artifacts. We also magnified all the images with a 4x factor. The original image, shown in Figure 7, has no blocking artifacts nor significant noise. After the DXT5 compression (Figure 8), severe blocking can be seen. Our algorithm attempts to mitigate the problem through the noise dithering operation. In Figure 9, the blocking artifacts are reduced, at the expense of adding noise.
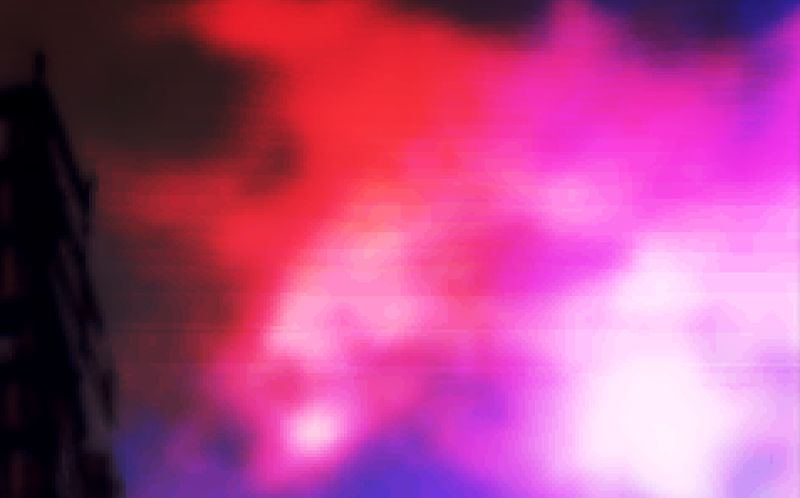
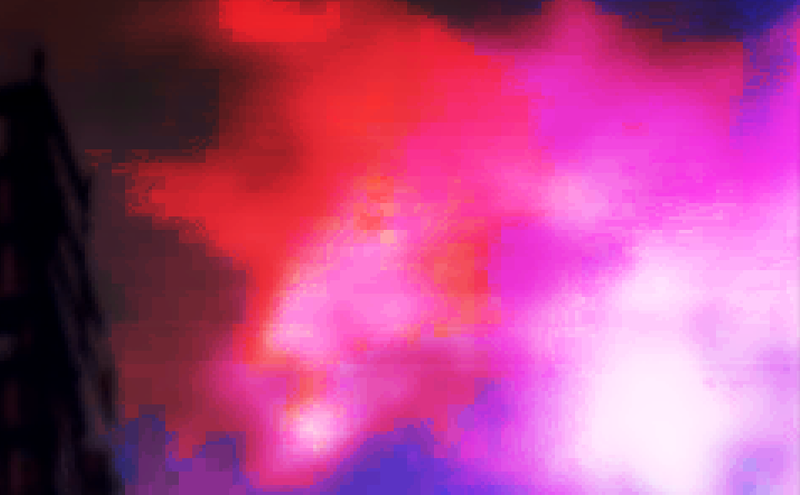
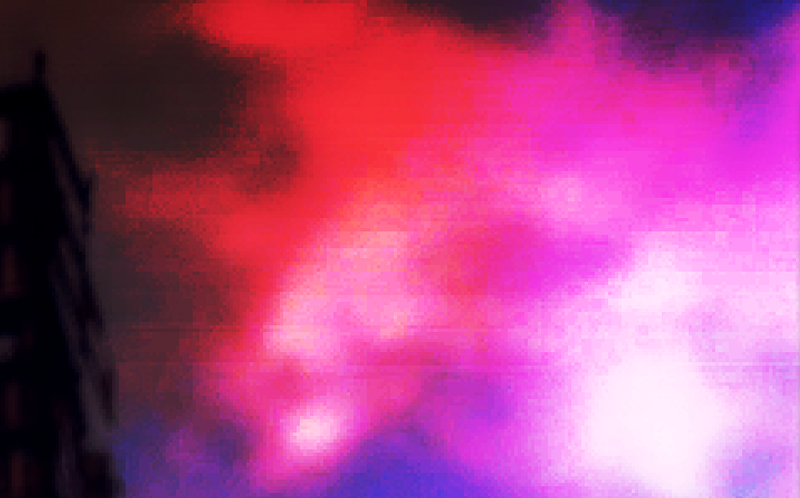
Removal of Banding
The following example set illustrates how banding is eliminated for the compressed image. Figure 10 is the original image. The sky consists of a smooth gradient with orange and yellow tints. After the DXT5 compression (Figure 11), banding can be seen from the orange and yellow part, and the gradient is no longer smooth. Using our method (Figure 12), the sky does not have any banding, and it looks identical to the original image. The removal of this artifact is contributed by the added precision in the chroma channels from the non-linear remap.
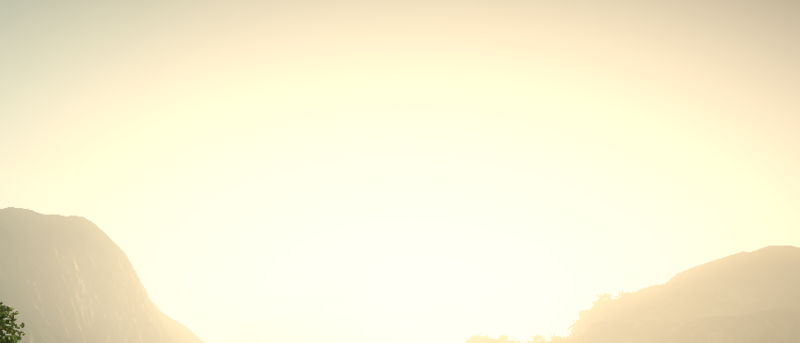
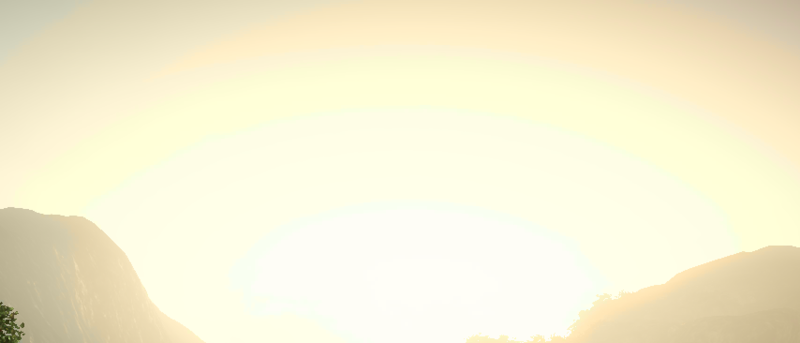
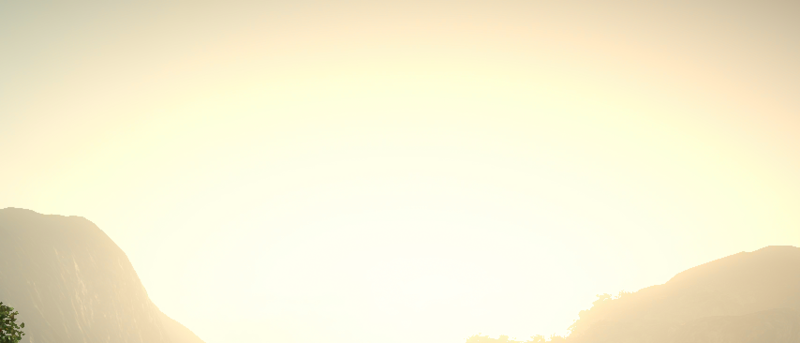
Conclusions
The improved algorithm effectively diminishes the banding and blocking artifacts caused by the traditional DXT5 algorithm through adding noise. The micro-sharpening adds back some fine details which are lost during the compression. And the non-linear remap minimizes some of the negative effects of the quantization. Overall, a higher quality image is produced without compromising the compression ratio.
The next step is to extend the current work to a non-real-time compression algorithm. The current algorithm is suited for real-time, which has a strict performance constraint. An extension to non-real-time work would relax this constraint and allow a more elaborate algorithm that works for offline image processing.
References
[1] http://en.wikipedia.org/wiki/S3_Texture_Compression
[3] http://wiki.multimedia.cx/index.php?title=YCoCg
Appendix I - Code and Data
Data - File names containing "base" or "original" are the original images used for testing. File names containing "DXT5" are the compressed results of the YCoCg-DXT5 algorithm (State of Art). File names containing "Fei" are the compressed results using the method in this paper.