Dual Fisheye Image Stitching Algorithm to 360 Degree Photos: Difference between revisions
Navi-Maggie (talk | contribs) |
Navi-Maggie (talk | contribs) |
||
Line 47: | Line 47: | ||
== Appendix == | == Appendix == | ||
=== Appendix 1 === | |||
=== Appendix 2 === |
Revision as of 09:11, 18 December 2023
Introduction
360-degree cameras provide utility by capturing all angles around the camera, or 360 degrees horizontally and 180 degrees vertically. This is different than the standard camera in your phone where the wide angle covers somewhere between 110 to 60 degrees, or a focal length of 10 to 25 mm [1]. There are many use cases to 360 degree cameras like virtual reality (VR) [2], generating digital twins for the purpose of cultural preservation [3], general photography, capturing events, and even virtual tours like Stanford’s [4]!
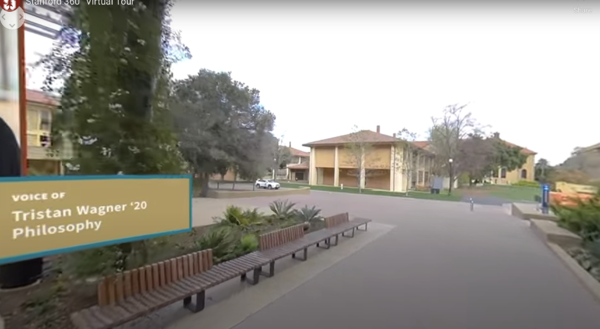
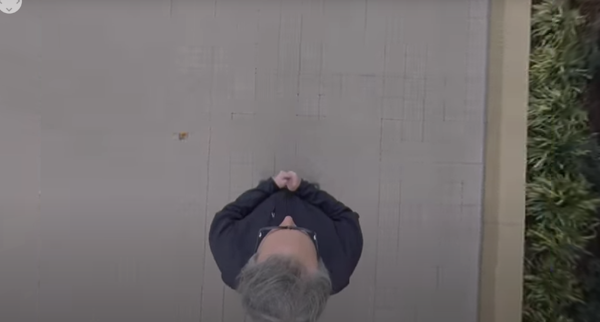
In Figure 1, you can see the Stanford virtual tour utilizing the 360 degree camera with the arrow symbol in the top left corner of the image. Additionally, they are utilizing editing features of the image to place notes to aid the tour. In Figure 2, you can confirm that the 360-degree video is indeed 360-degrees with the view of the man’s holding the camera’s head included in the tour.
There are two main types of 360-degree cameras: catadioptric and polydioptric [5]. Catadioptric cameras combine a lens (dioptric) and a mirror (catoptric) to take a 360-degree image. These types of cameras require calibration and are much larger as well as much more expensive than other types of 360-degree cameras. However, this type of camera geometry does not have as much camera parallax in the final image since there is not combining of multiple different images. Polydioptric systems involve stitching together images from multiple different wide-angle cameras. With these systems, the separate wide-angle, or fisheye lens, cameras have overlapping field-of-view’s so that the images are then able to be stitched together based on this overlap. The polydioptric systems are significantly more economical than the catadioptric systems, but do suffer from more camera parallax due to the required stitching in software.
The range of quality in different 360-degree cameras is large, as well as their cost. With the lower quality 360-degree cameras, more post-processing in software is required to avoid camera parallax. Something to note with the cheaper 360-degree cameras is due to their smaller contruction, there is significant vignetting across images. There, most likely, will be vignetting across most major 360-degree cameras before post-processing due to their wide aperture allowing light in at acute angles. However, this allows the technology to be more accessible to a larger audience. In this paper, the Samsung Gear-360 camera is used due to the widely available fisheye data online in addition to its lower cost.
Background
Since the Samsung Gear-360 is a polydioptric camera, software techniques for converting the fisheye lenses to one contiguous image will be discussed in this section. The specific camera in question has two fisheye lenses that are processed in software and joined to become one 360-by-180 degree image. Since each fisheye lens has a field-of-view of a little over 180 degrees, the two fisheye lenses will have some overlap that can be used to “stitch” the two images together.
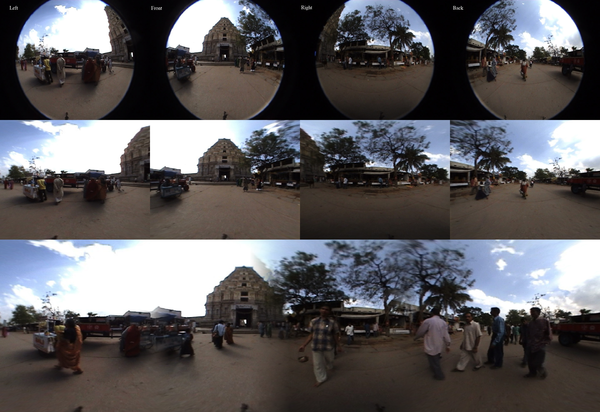
As seen in the top row of Figure 3, the image starts off being in a fisheye lense with some significant warping. Then, as seen in the middle row of Figure 3, each image is projected onto an equirectangular plane based on the assumption that the fisheye lenses keep the image in a spherical coordinate system. Lastly, the images are “stitched” together to generate one image.
As seen, the separate images in row 2 of Figure 3, have overlap across each one. This does introduce camera parallax as seen in row 3 of Figure 3. However, these overlapped features can be used to identify where stitching can occur. This can be done in a variety of ways including using machine learning technologies to complete feature matching across the different images, or the cross correlation to determine where the overlap occurs across individual images [5]. Another common way of determining the overlap is by using a homography matrix.
Lastly, there is a significant vignette on these images. To remove the vignette, post-processing in software can be used to remove the vignette including machine learning techniques and simple software techniques if the vignette model is known.
Methods
Results
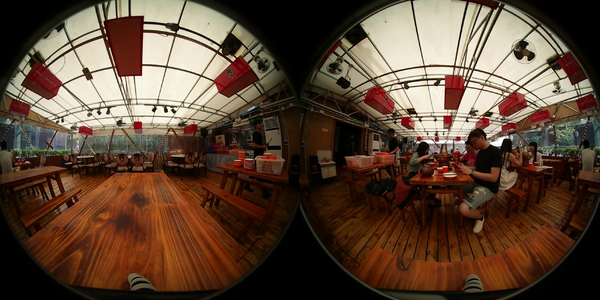


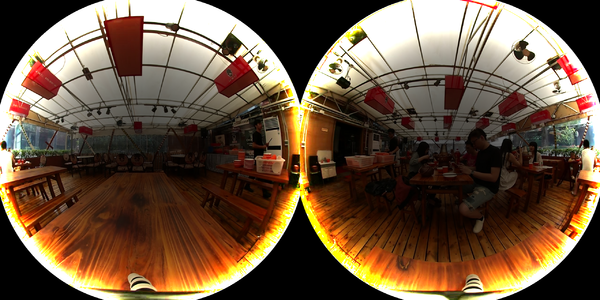
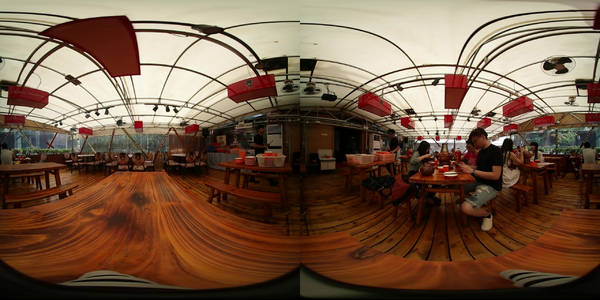
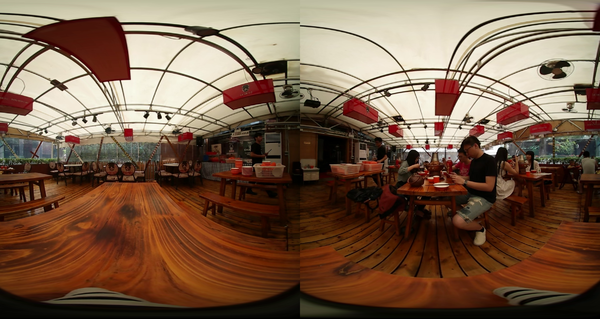
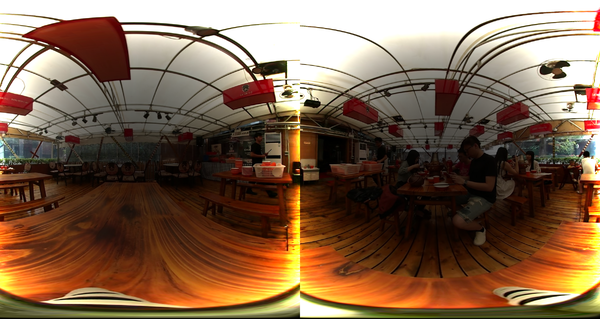